Put Your Data To Work
Datalytix
Revolutionize your business with Datalytix: AI-driven insights, drone and 360 monitoring, and innovative solutions like Optic and AVA for any industry.
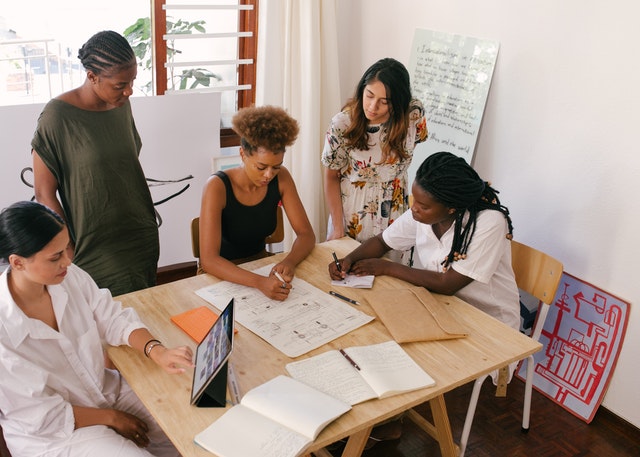
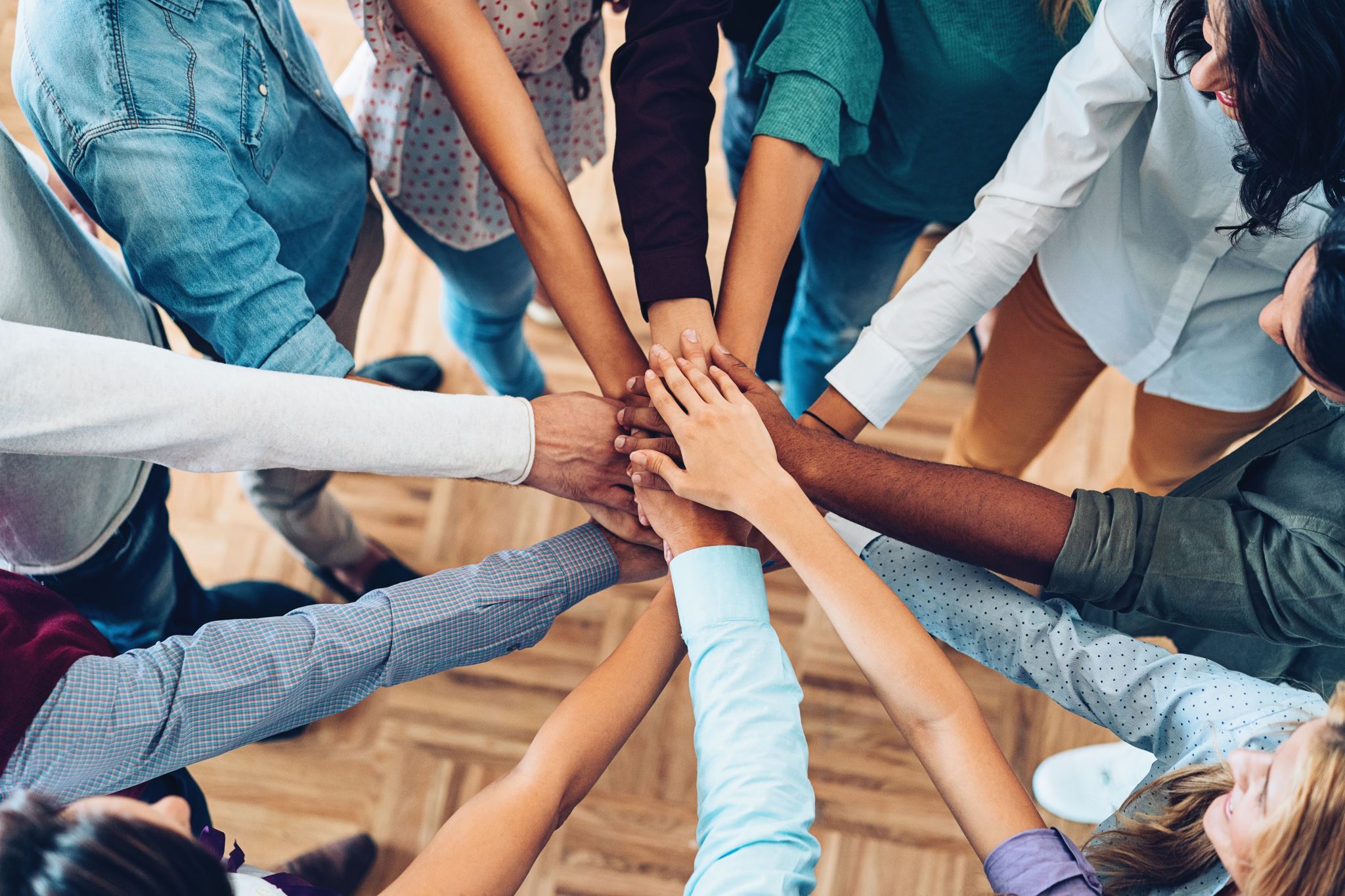
Who We Are
Datalytix is a team of passionate data and technology experts delivering AI/ML-driven solutions across construction, healthcare, and government sectors. Our tailored solutions help businesses optimize performance, mitigate risks, and drive innovation.
We Solve Real Problems
what can we do for you?
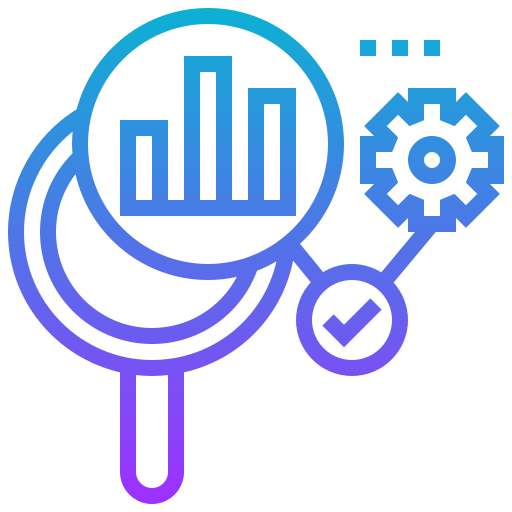
Advanced Analytics & Visualization Solutions
Leverage AI-driven intelligence to solve complex challenges, empower people, and win in your markets.
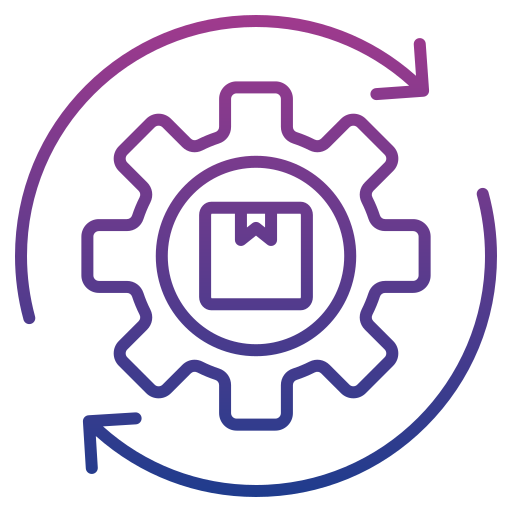
Analytic Process Automation (APA)
Automate and streamline resource-intensive processes. Put APA at the heart of your digital transformation.
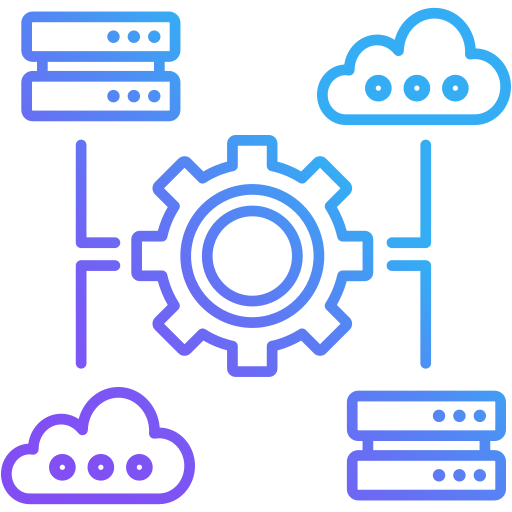
Data Management Solutions
Simplify collection, preparation, integration, processing, analyses, and management of data enterprise-wide.
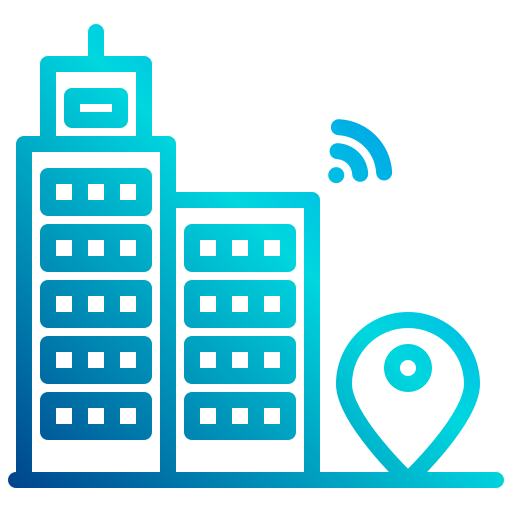
Location Intelligence
Harness the power of high-definition aerial maps that empower confident and informed choices
See how Datalytix works in fast-paced industries
Latest Blogs
What’s your toughest business challenge?
Schedule a complimentary 30-minute consultation
with a business analytics expert.
Join with our global community
Get In Touch
Address
Washington DC Metro Area
info@datalytixglobal.com